I'm moving !!!!!
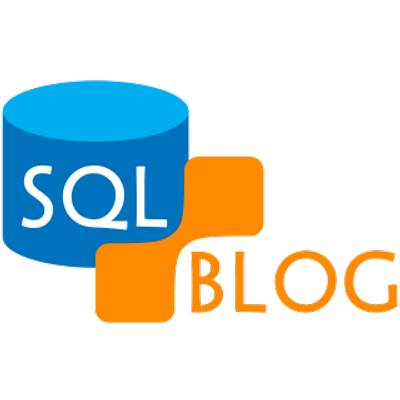
I am truly exited to move this blog to SQLblog.com !
http://sqlblog.com/blogs/maria_zakourdaev/default.aspx
You are more than welcomed to drop by.
Stay tuned
Over and out ;)
I am a Data Platform Microsoft MVP and a technology expert with more than 20 years of experience, a community leader with a profound knowledge of Microsoft products and services, while also being able to bring together diverse platforms, products, and solutions, to solve real-world problems. I have a hands-on experience managing various data management technologies in Azure, AWS and Google public cloud platforms, including SQL Server, Azure CosmosDB, AWS DynamoDB, MemSQL, MySQL, Postgres,Snowfla
Comments
Post a Comment